NEW YORK TIMES CHIEF DATA SCIENTIST CHRIS WIGGINS ON THE WAY WE CREATE AND CONSUME CONTENT NOW
"WHAT WE’RE AIMING TO DO IS TRY TO INFORM PRODUCT DECISIONS AND MARKETING DECISIONS, AS WELL AS POTENTIALLY NEWSROOM DECISIONS.”
The New York Times turned 163 years old this year. And while it might be understandable for an institution older than almost anyone not named Yoda to be less-than-cutting edge in its digital approach, that didn't stop the knives from coming outwhen an unflattering innovation report leaked earlier this year.
The next report will likely be different. That's partly because earlier this year, the Timeshired biologist and machine models expert Chris Wiggins, an associate professor of applied mathematics at Columbia University, as its chief data scientist.
Wiggins participated in a talk at GigaOm’s Structure Conference in San Francisco last month about how data science works, how it is helping to change the Times, and why he believes data literacy is essential for news-gathering companies and contemporary global citizens alike. Wiggins is far from alone in this thinking: new operations by upstarts such as former Timesman Nate Silver's FiveThirtyEight and ex-Washington Post reporter Ezra Klein's site, Vox--not to mention the Post itself, which just this week launched a new data-driven initiative called Storyline--have caused some observers to dub this the "wonk wars." The Times has its fair share of competition.
“At The New York Times, we produce a lot of content every day, but we also have a lot of data about the way people engage with that content,” Wiggins says. “[The Times] wanted to build out a data science function not only to curate and make available those data, but to learn from those data. In particular, the thing that the New York Times is interested in learning is: what makes for a good long-term relationship with a reader?”
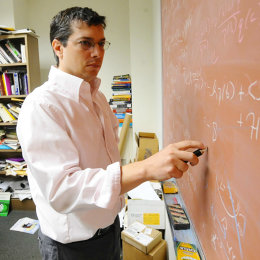
Though Wiggins is careful to point out that decisions are made first and foremost based on journalistic intuition, guiding decision-making is exactly what Wiggins’s work is doing. And according to some data journalists, that is long overdue. In an interview appended to Alexander Howard’s 144-page report on “The Art and Science of Data-Driven Journalism” Aron Pilhofer, previously at the Times and now theGuardian’s first executive editor for digital, puts it bluntly:
Right now, many newsrooms are stupid about the way they publish. They’re tied to a legacy model, which means that some of the most impactful journalism will be published online on Saturday afternoon, to go into print on Sunday. You could not pick a time when your audience is less engaged.It will sit on the homepage, and then sit overnight, and then on Sunday a home page editor will decide it’s been there too long or decide to freshen the page, and move it lower.I feel strongly, and now there is a growing consensus, that we should make decisions like that based upon data. Who will the audience be for a particular piece of content? Who are they? What do they read? That will lead to a very different approach to being a publishing enterprise. Knowing our target audience will dictate an entirely differently rollout strategy. We will go from a "publish" to a "launch." It will also lead us in a direction that is inevitable, where we decouple the legacy model from the digital. At what point do you decide that your digital audience is as important--or more important--than print?
While Wiggins is more circumspect, he offers excellent insight about how data science is changing the business of news--at the Times and beyond. He also explains why you should learn data, and why he is spending this summer teaching a new crop of data journalists.
Here's what he told us, onstage and off.
When It Comes To Content, What Should You Count?
“There’s this famous quote from Einstein that ‘Not everything that can be counted counts and not everything that counts can be counted. Number of clicks is very easy to count, but that’s not what counts at the New York Times.
We’ve built predictive models for many things, but one of the predictive models we built early on was a predictive model to see can you tell when a subscriber is likely to cancel their subscription.
We’ve looked at obvious metrics for engagement (some of which turn out to be predictive and some of which don’t): the importance of our comments, data about which parts of the site people engage with, how important is it that people engage regularly? It’s early days, but I would say that what we’re aiming to do is try to inform product decisions and marketing decisions, as well as potentially newsroom decisions.”
How Is Data Currently Being Used In The NYT Newsroom?
“I would say newsroom analytics at the New York Times is an active although early effort. It’s been active for about 14 months, which means it’s old enough to walk but not necessarily old enough to talk. There is definitely an interest in metrics. There’s also a strong interest in what’s called audience development: how do you make sure that not only do you create great journalism, but that journalism gets to as many readers as possible?”
I’m sure you’ve read the innovation report. If you haven’t read the innovation report that was leaked, I urge you go check it out. It’s this 96 page document, very honest and introspective, asking, among other questions, how do we use metrics in a way that promotes quality rather than simply trying to maximize quantity in a way that might threaten news judgment.
Even in the innovation report, they talked openly about A/B testing on which thumbnail image is associated with a picture. I don’t think it’s beyond the pale for the New York Times to consider, say--and this is a speculation on what the newsroom will decide--if you have a set of headlines that have all passed muster for satisfying news judgment and being sufficiently Timesian, you could imagine the Times potentially A/B testing on headlines. Not that they are. Not that anybody’s told me they will. I get the impression from people on the newsroom side that they are sufficiently data-curious that . . . That’s something you can do without violating any sense of news judgment.”
What Is The Future Of Mobile At The Times?
“Some of the things we do about predicting engagement with the new apps are very . . . are of interest to people in the newsroom.
The way people engage with content is very different on triple dub [the worldwide web] than on the mobile apps. The New York Times just built two new mobile apps, and they’ll be launching another. There’s a new mobile app called NYT Now and an Opinion app.
The way people engage with that is very different than the way they engage with the desktop. It’s a completely different experience in terms of how they explore content, the timing of when they use it, how long the sessions are. The features that turn out to be predictive of engagement on triple dub may be very different than the features that turn out to be predictive for mobile engagement.”
Why Should We All Be Data Literate Now?
“In order for there to exist critical literacy--the ability to take apart somebody else’s argument based on the way they analyze data--you need for there to be enough people who are savvy and able to use the data, to make sense of the data.
In the same way two different reporters might find a source and ask totally different questions and come to different interpretations, two different data journalists might encounter the same data set and have a variety of ways of trying to learn from those data. You really need there to be enough people doing data journalism for there to exist some sort of peer review.
When you’re reading somebody else’s data journalism, you really need to think through what assumptions were made in that model or in that analysis.”
FiveThirtyEight had done [an] analysis because there was this very important story from Nigeria about girls being kidnapped. They said, "Well, can you really see the extent to which there may be increases or decreases in kidnapping?" The way they analyzed this was to use a database called GDELT, which is really a database of new stories. There’s a couple problems there. But the most glaring is: that’s not necessarily and accurate listing of kidnappings, it’s just an accurate listing of stories written in the West about kidnappings. There are also uncertainties because part of what they did in this analysis was to actually plot out where the kidnappings were. But a lot of times, if people don’t know precisely within a country where it is, they’ll just locate it in the capital.
In general, whenever you’re analyzing a data set you really need to think about: how was it created, what biases or assumptions went into creating that data set; In the same way, when you’re reading somebody else’s data journalism, you really need to think through what assumptions were made in that model or in that analysis.
And of course, we can’t do that if we don’t have a citizenry and a group of journalists who are sufficiently literate in algorithms and analysis to be critically literate.”
What Tools Does The Times Use For Data Analysis?
“The device that you’re using for Facebook is also a potent device for weapons-grade statistical software, if you choose to use it for that. There’s nothing to stop you from using your computer as a tool not just for sending an email, but for [looking at] the data that are impacting decisions, policy, politics.
In 2014 it is no longer the case that there’s some great financial and technical barrier that prevents ordinary citizens from using state-of-the-art tools. Data analysis has gotten to the point where you can do such powerful analyses, statistically, from just anyone’s laptop. And open source and open suite software has gotten so advanced that the same weapons-grade statistical software that Google uses you could use, for free on your laptop.
For example, there’s an open-source program that’s called R. It’s very popular at Google, and it’s free. Anyone can use it. Similarly there’s an open-source language called Python, and there are some fantastic statistical analysis tools in Python that are free and anyone can use them. In fact, those are the same tools that we use in my team at the New York Times. They’re the same tools that are used at the most advanced technology companies. And those tools are available to any citizen.”
How Is Wiggins's Program At Columbia Moving Data Journalism Forward?
“The journalism school at Columbia is very forward looking. With the help of the Tow Foundation, [we’ve] created this new program called the Lede to try to train what we all believe will be the future generation of data journalists.
There’s a four-class summer program that I’m a part of to introduce people to: the basics of computation; the basics of algorithms, including exposure to machine learning ideas and data science ideas. And they’re really learning how to code and really learning how to use the computer as a tool.
The ability to use the technical tools, that’s part of it. But part of it also is critical literacy--to be critical about somebody else’s story that they’re trying to tell you using data. And rhetorical literacy which is the skill of being able to use data use data and analysis of data in order to make a point--in order to tell as story yourself. We’re trying to teach all of those things.
At the Lede we have a very diverse teaching body that comes at data from very different perspectives. There are four professors in all, people with PhDs in history, pure mathematics, and English. It’s a really diverse group, the four of us that are trying to teach this program.
What puts us in common is a set of values and the bravery to do it, not necessarily our PhDs or the field in which we’re trained. I think you see multidisciplinary groups form when there are new ideas that are not yet captured in any existing discipline."
[Image: Flickr user Martin Fisch]
No comments:
Post a Comment